Our Solution
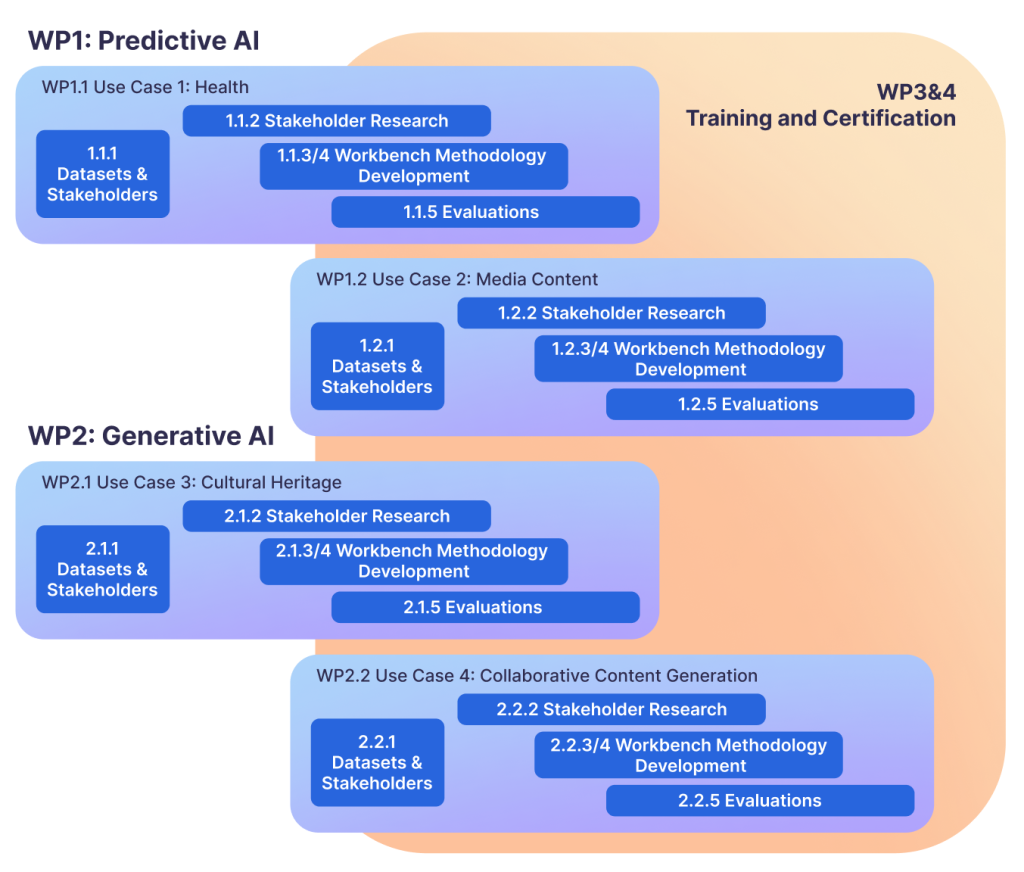
We will start each use case with acquiring datasets and gaining access to stakeholders. We will identify any gaps in stakeholder representation, and work with ‘gatekeepers’ to invite stakeholder to participate in our research activities. We will gain access to confidential datasets, which might require non-disclosure and collaboration agreements. We will produce synthetic datasets if we cannot access confidential datasets.
Stakeholder research, supported by our partners, will use interviews, co-creation workshops and situational observations with relevant stakeholders. Using thematic and semantic analysis of this data, we will uncover stakeholders’ perspectives on potential harms, fairness notions, ways of assessing fairness, and ‘baselines’ for auditing AI. Leveraging existing prototypes from our project team’s prior work, such as FairHIL and ArticlePlaceholder as starting points, we will extract requirements for workbenches.
The findings of our stakeholder research will be used as input to develop workbenches to support stakeholders to audit AI models. In the predictive AI use cases, we will focus on developing new measures that better capture and prioritise stakeholders’ notions about harms, which will be integrated into the auditing workbenches. Further, we will explore multi criteria decision-making and optimisation approaches to manage the trade-offs between measures, in both models and their underlying datasets using stakeholder audit feedback as ‘signals’ for trade-offs. At the same time, we will develop privacy-preserving mechanisms that, while allowing an audit, protect personally identifiable information and sensitive information contained in datasets and models, leveraging previous work in e-voting audits and countering inference attacks involving protected characteristics. In Use Case 2, we will consider how notions of fairness in search engines vary from query-to-query, and across different stakeholders, in a multi-lingual and multi-modal deployment. For Use Cases 1 and 2, we will design and develop user interfaces (UIs) suitable for a range of stakeholders, exposing model information to help in assessments as well as obtaining user feedback from lay users. We will pay particular attention to explaining AI concepts and measures to non-technical users, as well as supporting them to audit the AI models and underlying datasets. The UIs will also integrate new measures, multi-criteria decision-making and optimisation and privacy preserving mechanisms. We anticipate that workbenches for generative AI might require alternative capabilities. For example, for Use Cases 3 and 4, we will focus on developing methods which provide explanations and chain of thought reasoning/steps of generated content, to provide transparency and accountability of generated content. Moreover, we will develop new measures to quantify the groundedness of generated content. Further, we will explore the accuracy of the inferences made by the generated data and whether there are any systemic biases or stereotypes in such inferences which may result in unfair and harmful assertions. We will expand the multi-criteria exploration of these new measures, considering prompting strategies relevant to generative AI. This will lead to the development of novel user interfaces, which will provide accessible information about what is happening ‘under the hood’ to stakeholders and allow technical processes to be ‘readable’ by the stakeholders. Feedback from users to steer the model to provide better annotations, explanations and expansions will be obtained and incorporated via the UI. The workbenches will also guard against and potentially mitigate biased interpretations where actors try to introduce or manipulate the system to “prove” or “slant” the historical evidence to fit preconceived narratives or doctrines. In Use Case 4, we will extend the ArticlePlaceholder tool with bespoke capabilities to validate and improve AI-generated text and references, access explanations centred on the provenance of information, and investigate and enhance the underlying data. By updating newer generative AI models with finetuning datasets, we will be able to include dataset auditing in the workbenches, besides model auditing. Moreover, auditing is a collective task. Hence, we will use computational argumentation techniques, building on our prior work to support auditors in making explicit their reasons and mitigating any groupthink or authority bias, as part of developing novel algorithmic approaches that guard against harm by malicious actors introduced through their feedback during participatory auditing.
Hand in hand with the workbenches, we will develop participatory auditing methodologies. As part of each use case, we will review regulatory frameworks in the UK, USA, Japan and the EU and interview policy and decision makers to embed findings in auditing workbenches and the AI development lifecycle. Informed by existing work in AI assurance, we will run co design workshops towards a participatory auditing approach using the workbenches we develop, to further address social biases in how collaborative decision-making during audits is carried out. We will investigate the legal implications of using the auditing workbenches e.g. copyright and licensing. We will also investigate how participatory auditing using collective feedback can be scaled up, how to effectively ‘sample’ auditors to represent stakeholders, how to give voice to stakeholders without a background in AI, and where in the development lifecycle to integrate participatory auditing.
We will rigorously evaluate the workbenches and methodologies through prototype-driven experiments in each use case. We will work together with partners and stakeholders using a mixed-methods approach. We will invite relevant stakeholders to apply the auditing methodology and workbench to audit the AI systems. We will record and analyse stakeholders’ interactions with the participatory auditing prototypes, and capture qualitative user-centric data, such as subjective workload ratings, subjective usability experiences, trustworthiness, performance and fairnessratings as well as quantitative measures for accuracy, precision, recall, bias and fairness, especially using EDI dimensions to ensure fair decision-making. In addition, we will employ quantitative measures, including Bilingual Evaluation Understudy (BLEU) score, perplexity, generation speed (latency), fluency, and factual correctness. We will collect qualitative feedback about the participatory auditing methodology. The continuous, cross-cutting evaluations will allow us to compare between use cases, draw out context-specific workbench and methodology guidelines and make workbench and methodology improvements across the project.
Project activities will also involve developing training and certification. Distilling our research findings across all use cases, we will develop training materials for stakeholders in participatory auditing, leveraging project partners Scottish AI Alliance’s Living with AI course and AI Playbook, and relevant training materials by partner Centres of Doctoral Training. Furthermore, we will work towards a certification framework for AI solutions. We will investigate possibilities for self-certification, which evidences that a participatory auditing methodology was employed, and quality standards have been achieved. We will look towards tying certifications into regulatory frameworks such as forthcoming UK AI regulation and the EU AI Act that govern the responsible development of AI.
Our project will directly engage with stakeholders and external organisations. We will offer awareness and training campaigns. We will also widely disseminate our findings to the academic community through publications at high-profile conferences applicable to this area. We will draw on Scottish Informatics and Computer Science Alliance (SICSA) to disseminate our work to other researchers. We will establish a network of stakeholders of existing partners, open to new members, meeting annually. We will disseminate our findings by participating in industry-focused events, such as Wikimania and the Scottish AI Summit.